With the expansion of the information age, contested domestic violence facilities, which have traditionally relied on secrecy in order to operate effectively, are now developing new models that bridge the gap between open invitation and secure enclosure, seeking new architectural methods that promote security, safety, and privacy, while also granting freedom, independence, and dignity.
The Urban Institute is a conjunction of varied programs that are centered around research, education, and advocacy, working to promote better quality of urban life through design. The institute uses various parts of the building as flexible rental space for social events and business purposes, posing a need for widely applicable designs that fit a wide range of needs and organize different levels of privacy.
The interior gallery space includes a variety of strategies that aim to limit user discomfort often caused by glare and thermal heat gain, sustainably source materials, and promote stimulating environments that guide the inhabitant through a variety of experiences.
Key elements include:
-Protected windows without exposure to extreme southern sun
-Adequate shading & light shelves to avoid internal glare
-Divided spaces to provide varied lighting conditions that guide the user’s journey through the gallery space
-Clerestory windows to let in subtle light, while avoiding harmful direct sun rays
-Outdoor gallery space to enjoy evening sunset lighting
This form serves as an abstract study of building components, focusing on the role of not only circulation, but the intersection of architectural masses and what spaces can be created in these moments. This is a case in which stairs move beyond the traditional sense of circulation and begin to create an intricate array of elevated experiences, become sites for highly varied programs, and serve a key aesthetic purpose that helps define space yet simultaneously complicate our perception of what one can occupy.

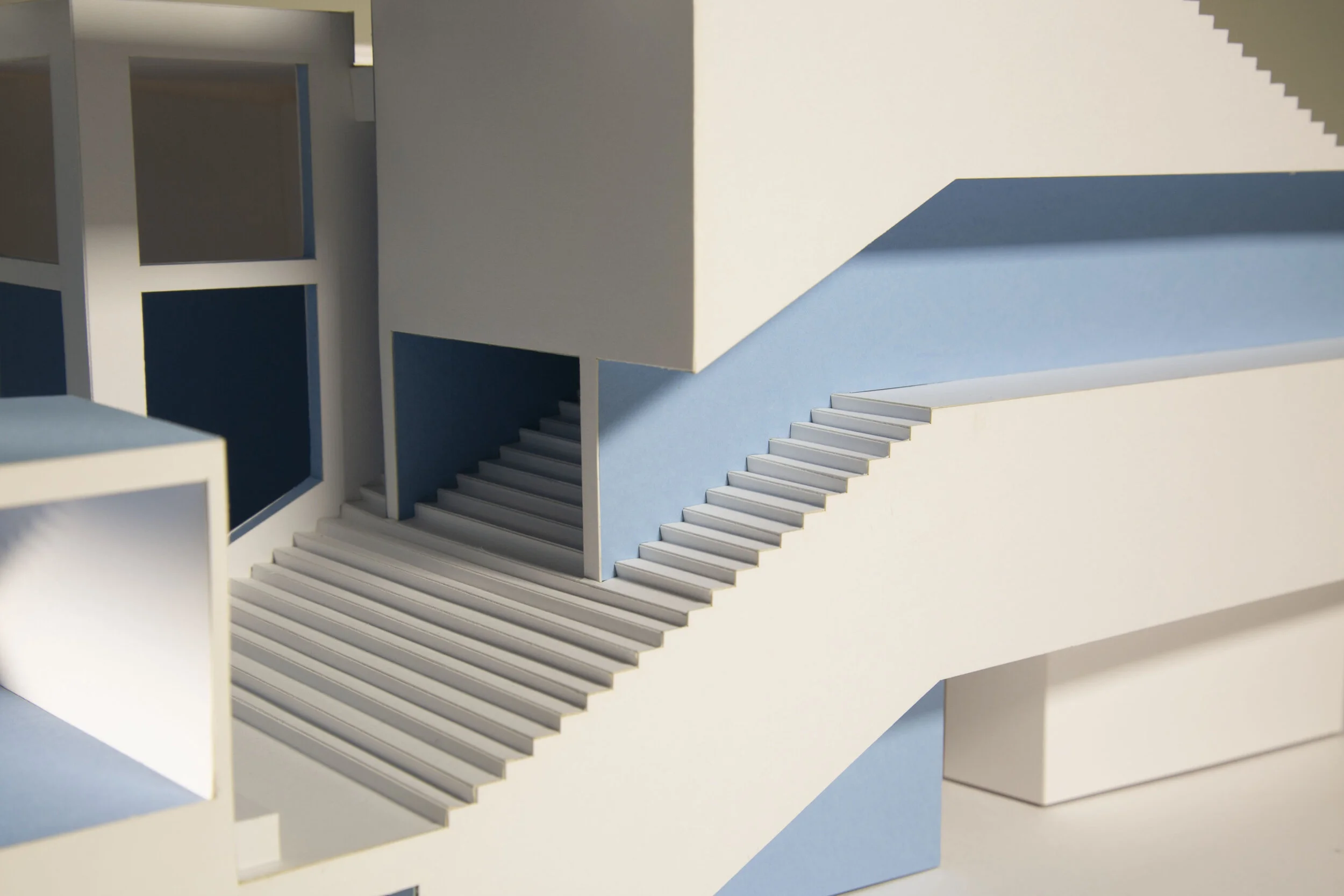
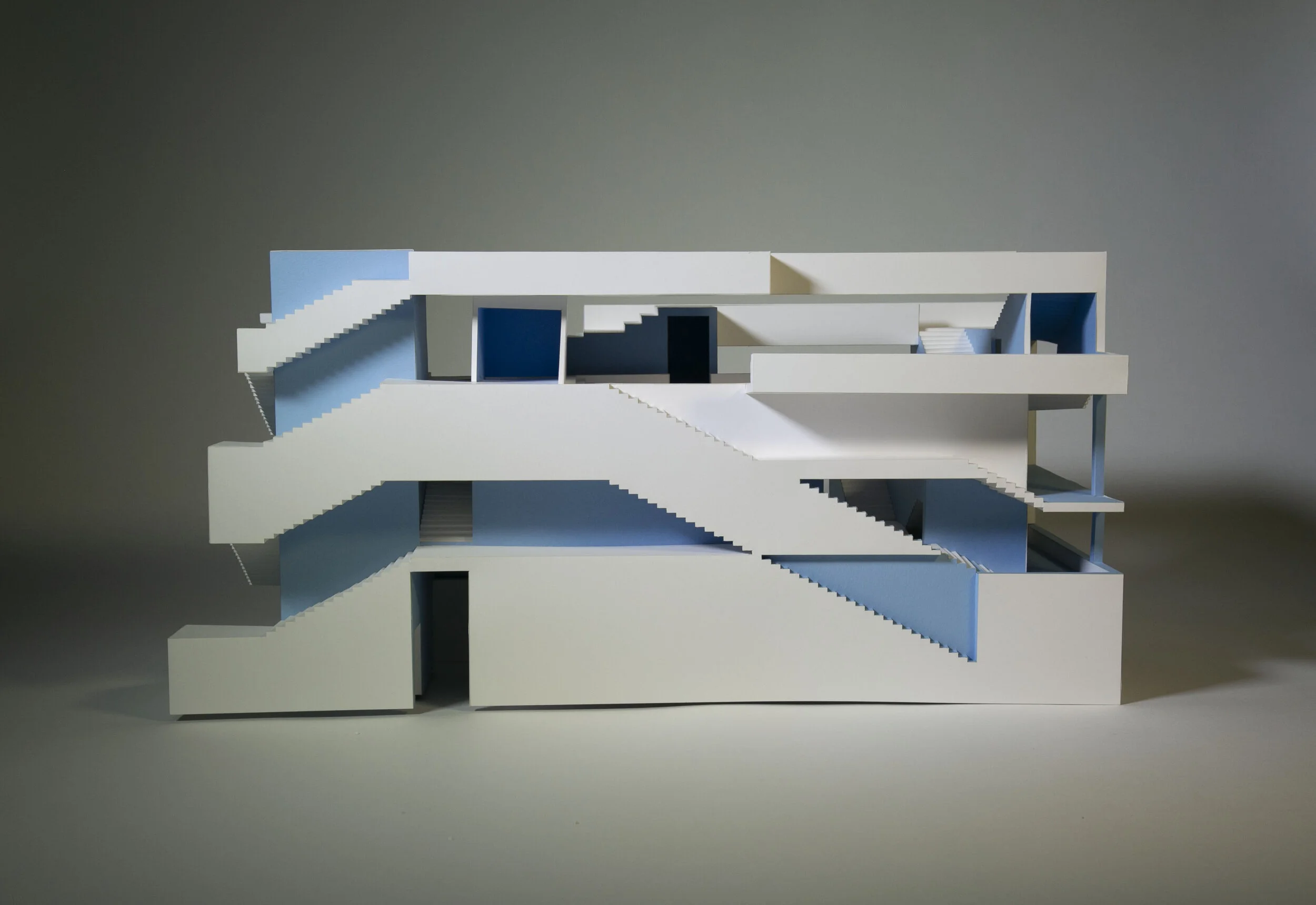



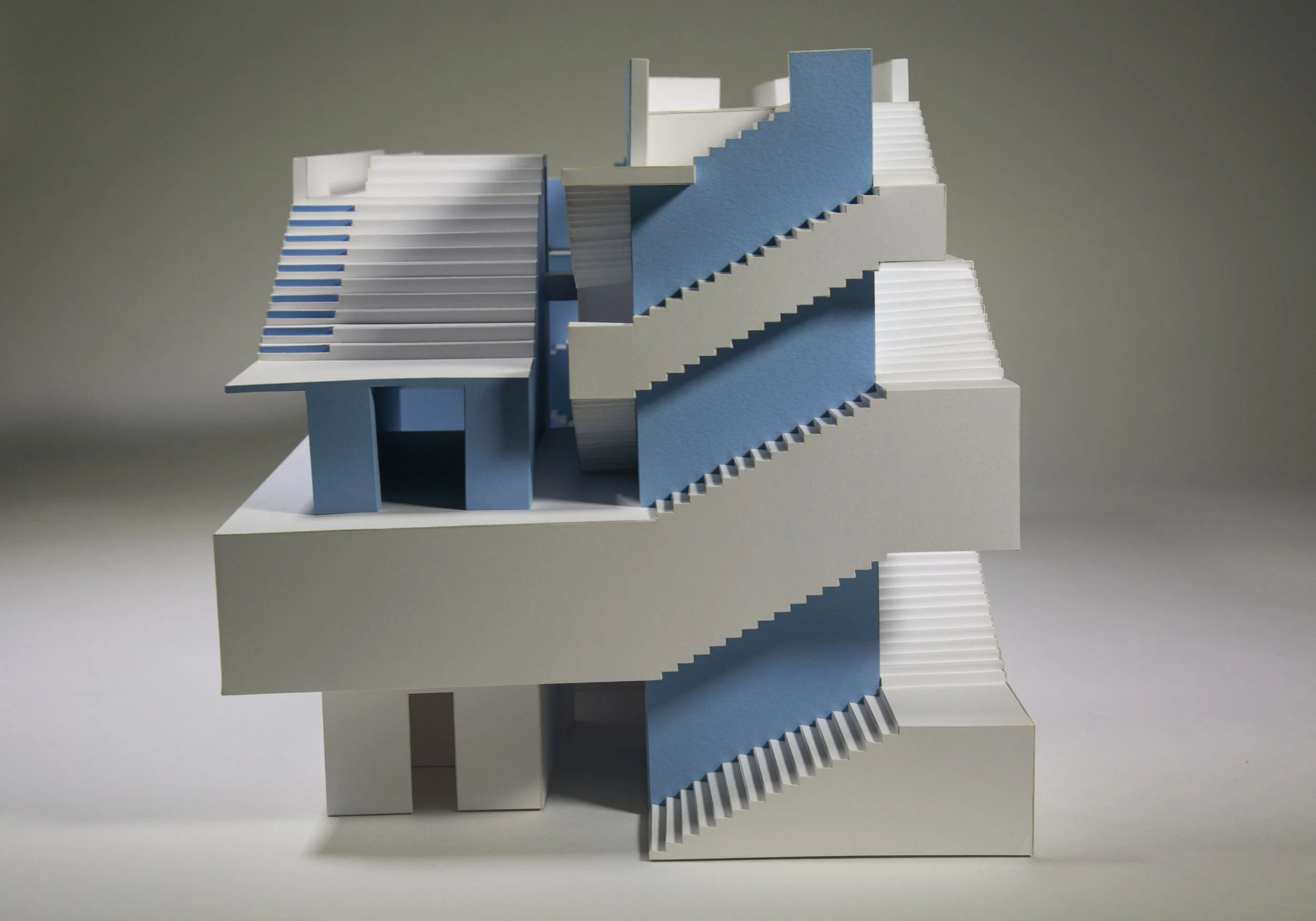
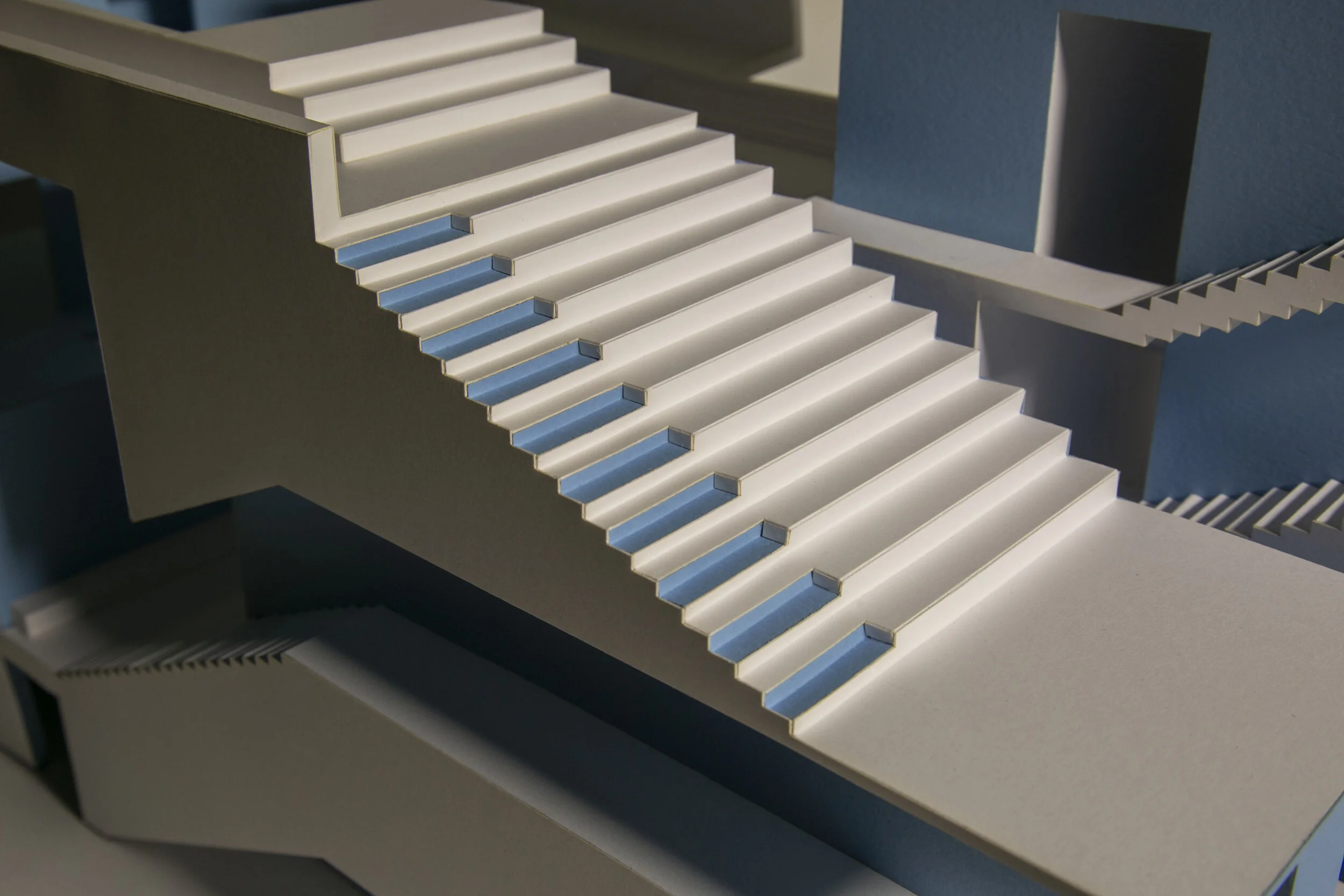
Process Photos…
Wondering what goes into making hand-made models like these? After building a life-scale digital model in Rhino3D, the “unrolling process” begins. This means scaling the digital model and deciding where to split up each surface or connect them for manageable “fold” and “score” lines: this is determined based on ease of connection in order to create the cleanest crease/corner edges. When fed into the laser-cutter (about 5 pages worth of the layout shown to the right), red lines are cut lines, blue lines are score lines, and green lines are personal mental maps (not used by laser cutter) to show where the reverse crease lines will lie. Then, the puzzle begins!
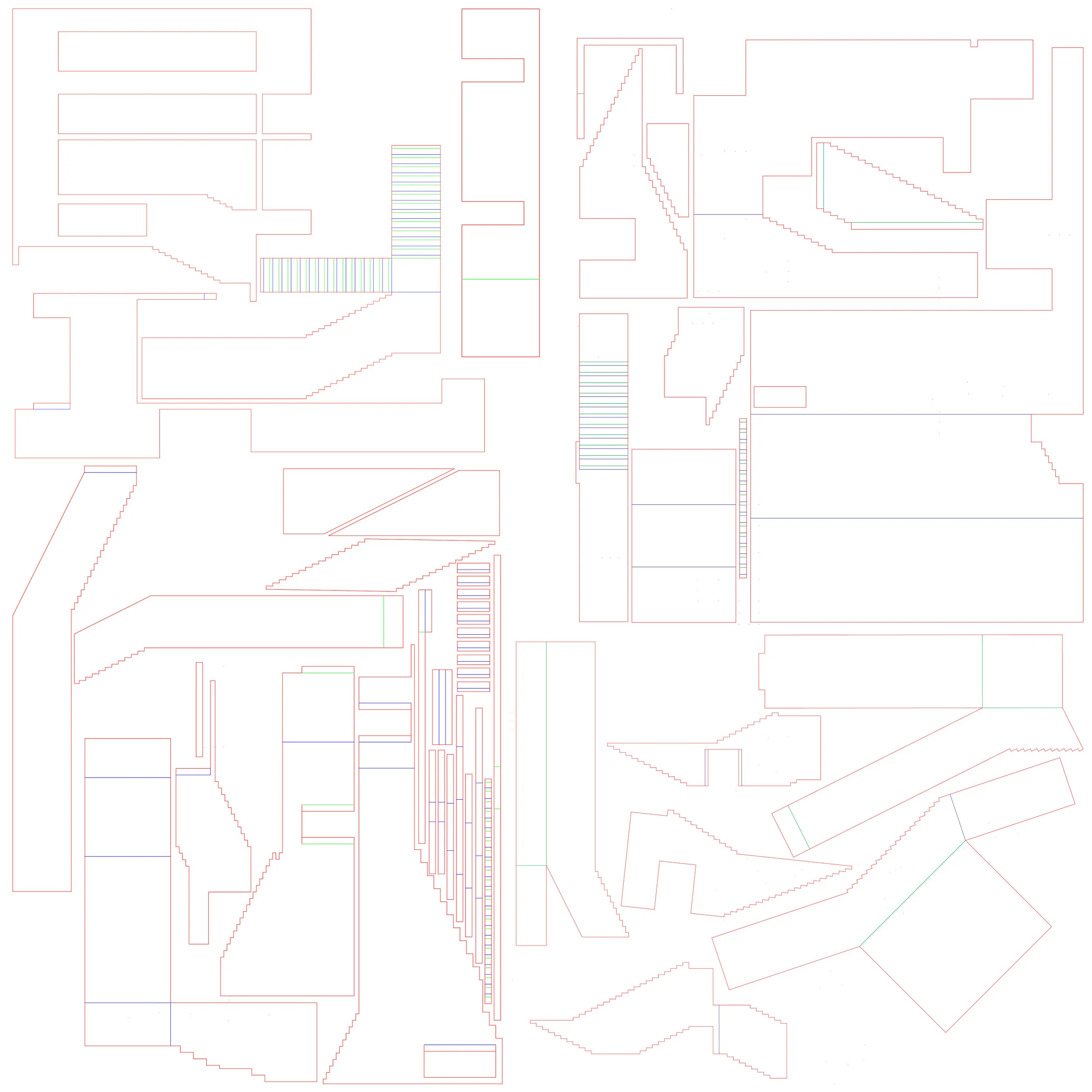

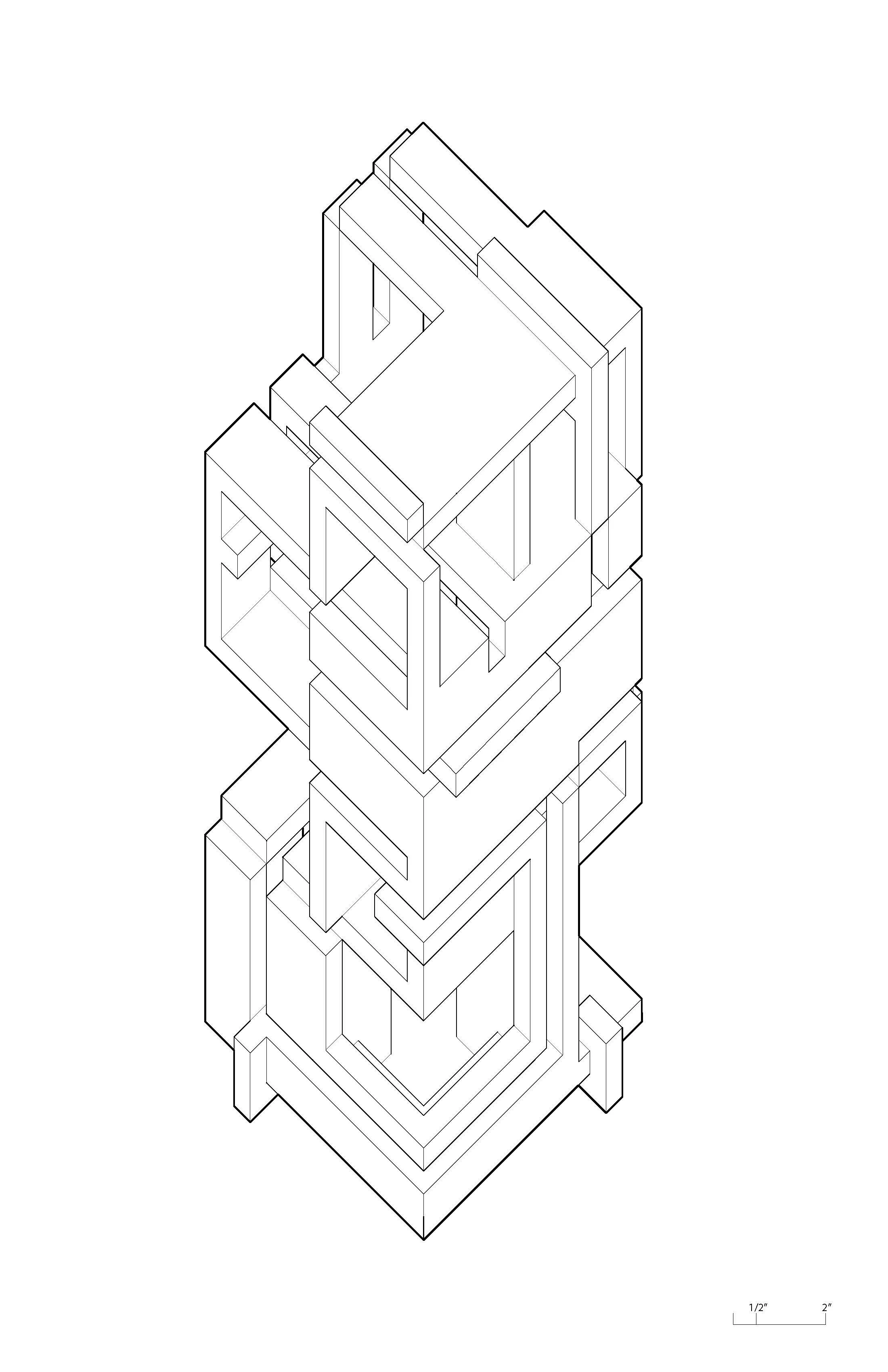
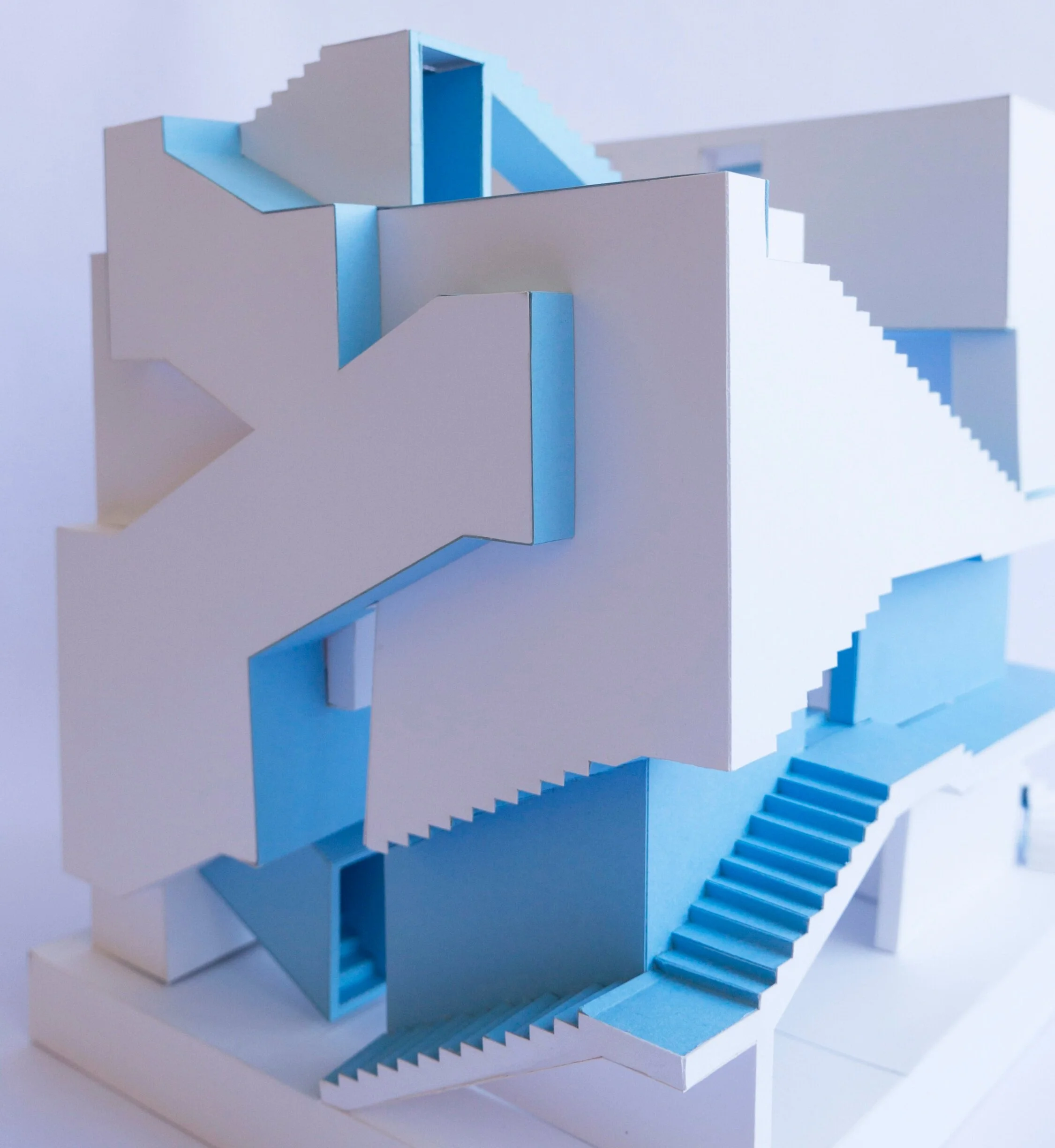


Serving as an exploration of the role of machine-augmented learning in the design process, this project is born out of a program called “Sketch2Pix,” which allows a user to program and train artificial intelligence to “look” at a rendered image and its corresponding (simplified) shaded line drawing thousands of times and then consequently recreate the rendered image in real-time when prompted by a manually, human-drawn shaded line sketch.
This study presents AI as a method of generating imperfect mimicries of traditional cultural symbolism, which leaves room for creative interpretation that stems from curves, cracks, splotches, and deformations that improvise on rather literal Greek and Roman symbols of strength, unity, and democracy.
Process:
1. Use Creaform 3D scanner/manually model target object with textures applied in Rhino3D.
2. Render ~2000 images (made up of trios: a render, a line drawing, and a monochromatic solid & void) using custom Python script, sheared & rotated at different angles.
3. Feed images through Google Collab, generating Python scripts for each object.
4. Upload Python scripts into Runway program, which is used in collaboration with custom “Sketch2Pix” Photoshop plugin.
5. Manually draw intended object, and trained model attempts to fill in the user’s intention with what it has “learned.”